5
score
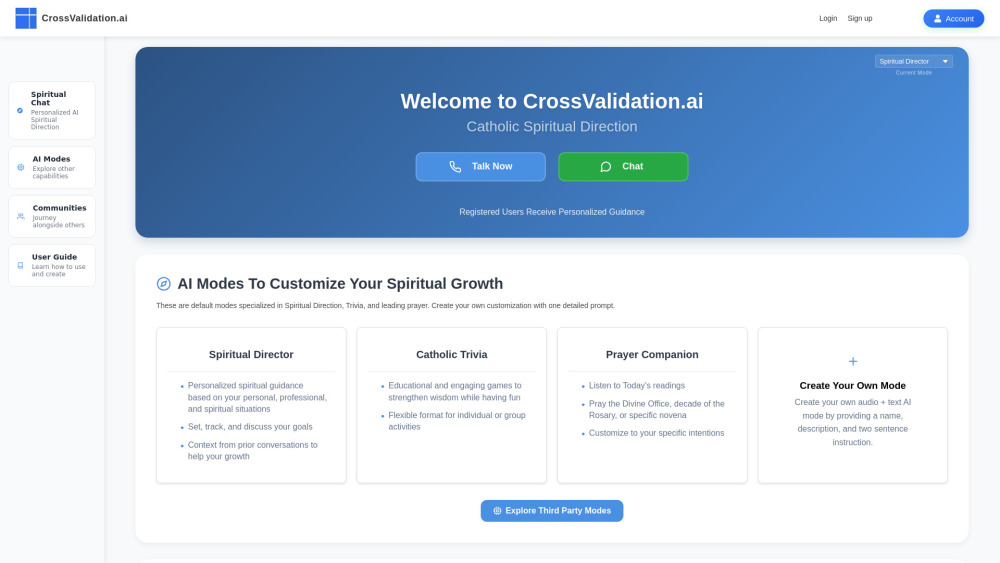
Simple Introduction CrossValidation.ai
CrossValidation.ai simplifies and enhances machine learning model validation with AI-powered automation.
Discover The Practical Benefits
CrossValidation.ai is an advanced AI tool designed to streamline and enhance the validation process for machine learning models. It automates various cross-validation techniques, ensuring models are both robust and reliable. The tool supports multiple validation methods, including k-fold, stratified k-fold, leave-one-out, and time series cross-validation. Users can customize validation strategies and parameters to suit their specific needs. CrossValidation.ai also provides detailed analytics and visualizations to help assess model performance across different validation folds. It integrates seamlessly with popular machine learning frameworks like TensorFlow, PyTorch, and Scikit-learn, making it easy to incorporate into existing workflows. The tool is designed for data scientists, machine learning engineers, and researchers who want to improve model accuracy and generalizability. Its intuitive interface and comprehensive documentation make it accessible to users of all skill levels. By using CrossValidation.ai, users can save time and effort while achieving higher-quality model validation results.
Perfect For
Improves model accuracy and saves validation time.
Machine learning engineers
Streamlines workflow with automated validation techniques.
Enhances model reliability with robust validation methods.
Key Features: Must-See Highlights!
Automated cross-validation:
Streamlines model validation with automated techniques.
Multiple validation methods:
Supports k-fold, stratified, leave-one-out, and time series.
Customizable strategies:
Allows users to define their own validation parameters.
Comprehensive analytics:
Provides detailed performance metrics and visualizations.
Framework integration:
Works with TensorFlow, PyTorch, and Scikit-learn.
visit site

FAQS
What types of cross-validation does CrossValidation.ai support?
CrossValidation.ai supports k-fold, stratified k-fold, leave-one-out, and time series cross-validation methods.
Can I customize the validation parameters in CrossValidation.ai?
Yes, the tool allows users to define their own validation strategies and parameters to suit specific needs.
Which machine learning frameworks does CrossValidation.ai integrate with?
It integrates seamlessly with TensorFlow, PyTorch, and Scikit-learn.